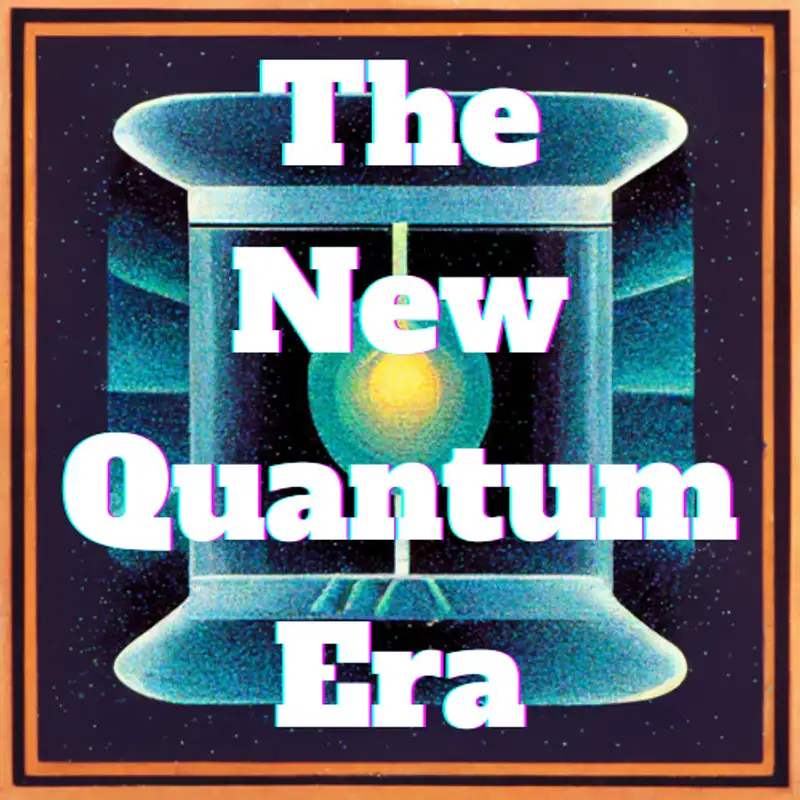
Qolab Emerges from Stealth Mode with John Martinis
Hello, and thank you for downloading episode 49 of the New Quantum Era. I'm your host, Sebastian Hassinger. Today's episode was recorded at the American Physical Society's Global Summit a couple weeks back with support from the APS who very kindly provided space where I could record these conversations away from the, the noise and bustle and, the continuous activity of the global summit. This episode is also brought to you with support from Quantum Machines Incorporated, is OPX 1,000 is tailored for large scale quantum computers offering unparalleled performance, scalability, and HPC integration, including an ultra fast interface to GPU and CPU accelerators for boosting quantum control. In this episode, I was joined by another of the critical pioneers of the field, John Martinez.
Sebastian Hassinger:John has a a storied academic career, but is probably best known for his work at Google where he led the effort to build a system that carried out the quantum supremacy experiment in 2019. It represented a a major milestone in terms of progress and also visibility of the field of quantum computing. I think for a lot of people, that's when quantum computing sort of got on their radar. John's been working on a new startup with cofounders Alan Ho and Robert McDermott called Colab. They recently came out of stealth mode, and John has made time to sit down with me to talk about Colab's goals and unique approach to quantum computing.
Sebastian Hassinger:I was so excited about the conversation with John. I kinda skipped over the usual intro, to an interview, so you'll hear me jump right into it. I don't think that, will be a barrier to enjoying the conversation, so let's hear. When we talked last, you were still in stealth mode. Right.
Sebastian Hassinger:And knowing that you were sort of out of stealth mode now, I was skimming back over that paper on, you know, building a scalable million qubit system.
John Martinis:Oh, yeah. That we published a few months
Sebastian Hassinger:ago. Right. Exactly. Exactly. Which is a great read.
Sebastian Hassinger:It's a really good, overview of the systematically sort of like what are all the go ahead.
John Martinis:So did you get through the whole paper? It's a long paper.
Sebastian Hassinger:It's a long paper. This morning, no, did not.
John Martinis:Yeah, that's okay. But what I like is it has, it talks about the whole stack and whatever you're interested in, you can read that section and figure out what's going on.
Sebastian Hassinger:That's
John Martinis:right. But the whole point of the paper was to really talk seriously about everything. Right. So that people can see that there's a plan. Obviously things change.
John Martinis:Yes. Okay, as you do that. But and also that we thought not just, you know, how to improve the qubits now, but how you go to a 10,000, a hundred thousand, a million. Right. Okay.
John Martinis:And what we're trying to do is build on the infrastructure and test it at a few qubits or whatever. Yeah. But it's gonna be upward compatible. Right. We're not gonna say, oh, we're gonna use integrated circuit fab.
John Martinis:You know, you have to go much deeper into that to make sure that it's really gonna work. Yeah. Yeah. So that was you know, that's what we've been thinking for quite a few years now, as you said, in stealth mode. And I didn't have the motivation to work on it until our collaborators worked on the whole stack and said, okay, it's really time to do that.
John Martinis:But yeah, that was kind of one of the nicer papers I've been on in my career, because it just pulls together all this thinking that we've been doing over the past decade.
Sebastian Hassinger:And what immediately occurred to me was something that you said in our conversation a couple years ago that that really stuck with me in that, you know, you have a a systems approach
John Martinis:Right.
Sebastian Hassinger:To the way you're thinking. You're not, you know, experimental physics gets you so far, but to build something with this kind of long term roadmap, there needs to be an architectural sort of philosophy and a systems design kind of approach. And that's really apparent in that paper.
John Martinis:Yeah, that's right. And thank you for noticing that because that's kind of what the big focus was. And again, you know, what is the long term vision? What do you have to build to do something? And then you back back away.
John Martinis:Now, I've been thinking about this for a long time, but the paper forced us to be real disciplined to communicate that properly. I'm glad it got across to you because that was kind of our intent and to do that. And the other nice thing, of course, now we're out of stealth mode, and I'll talk about it in my talk later today, is we have a very unusual business model in that we're collaborating with a lot of semiconductor companies. So typically what the various quantum computing people like Google is they're big enough, they can fund the whole stack. As a startup, as a lever, we can't do that.
John Martinis:So we collaborate with many people, and especially with many semiconductor companies who have really deep expertise in different areas. And that way you get to take advantage of that expertise. Specifically, they're going to be able to scale better because they know how to do. And they'll know how to keep costs down, which is like no one talks about costs for a quantum computer. And it's big.
John Martinis:But the hope is we can manage that a little bit better. Now, the hard part about this is you have to share and you have to collaborate. And you have to communicate to this whole team, you know, the details of what you're going to do. And that's kind of everyone kind of wants to hold their information tight and not share their infrastructure. But we decided that, you know, that's the best way
Sebastian Hassinger:to move forward. Now I'm getting where the name, Collab originates.
John Martinis:Yeah. That's really big Yes. That's you. Got it. And that was kind of the ethos that we started with.
John Martinis:Right. Is it, you know, that we And you know, this is not that crazy of a business because when you think about the computer industry, semiconductor industry, it was like IBM for many decades. And then in the '70s, and especially in the '80s, instead of everyone doing everything, all branched out and people did their different specialties. And there's a natural reason why that happened and it's better.
Sebastian Hassinger:Well, I mean that reflects the sort of systems thinking right from the beginning, right? You're anticipating this thing growing to a scale where one entity I mean, in IBM, even if it at its peak still had a supply chain. Right?
John Martinis:I mean That's right.
Sebastian Hassinger:There still is this sort of large, multiparty kind of collaboration that needs to come together. That's right.
John Martinis:Yeah. And I I would think that's the right model.
Sebastian Hassinger:Yeah.
John Martinis:And I we think we're far enough along that it's time to switch. It's like the seventies, you know, in the semiconductor industry. It's time to switch. We're trying out that model, but it's actually been working pretty well. That's great.
John Martinis:Like it a lot. It makes sense. And then, CoLab acts as system integrator at the low level hardware end. And then in our collaboration at the very high end, we have HP Enterprises, which makes supercomputers. So they're going to be the system integrator at the high end.
John Martinis:When you think about their expertise in software and running complex workloads, that's really good collaboration. We talk enough of the same language that we can do this.
Sebastian Hassinger:Yeah. And it's also, I think over the last couple of years has been a dawning realization that to put these devices, these eventual future devices into productive kind of solutions, there's gonna have to be very tight integration with classical computing at scale. So an HPE is a really good choice for that.
John Martinis:Yeah, HPE is good. And the other thing that happened is in our paper that's coming out, hopefully in a journal, NVIDIA is now on the paper. And that would be a very good collaboration because, of course, they make powerful GPUs. And I think if you want to do the error correction decoding, like, let's say simply and powerfully, then that's the way to do it. Now, still have to show you can do it.
John Martinis:It's not obvious. It's a hard problem. One of many. Yeah. And for example, like Riverlane, they're trying to build ASICs, which is maybe the right way to go, but it's certainly a good way to go.
John Martinis:Right. We do NVIDIA, and then, you know, we'll see what's
Sebastian Hassinger:What I noticed also you're partnering with quantum machines because you you call out in that paper at multiple sort of inflection points how the wiring and the control systems are going to be one of the major stumbling blocks for transitioning Yeah.
John Martinis:We kind of took it at every power attempt from a hundred to a 10,000, hundred thousand. We talk about what you need to do and how to think about that properly. And that was a very good way for us to kind of describe what's going on. So we have an integrated solution that scales to about 20,000 qubits. Wow.
John Martinis:And then, you know, which is a one module, and then you put together the modules by tiling them together, which can then scale up, you know, to a couple hundred thousand to maybe a million. And then, you know, that's a pretty big dilution refrigerator and maybe one
Sebastian Hassinger:thing So that's all in one fridge you're expecting?
John Martinis:Well, we think at least about 200,000 is one fridge.
Sebastian Hassinger:So you definitely have some new approaches to wiring and controls. Yeah, that's one big coax per cubit is not going to come out.
John Martinis:Well, actually it's a brute force wiring. Really? But if you use the proper semiconductor, you have to use semiconductor manufacturing. So we're doing wafer scale integration and then we're using flex for, not coax, but flex. People know how to make printed circuit boards.
John Martinis:Yeah. Okay? And then you use CMOS control electronic ASICs, which we need to investigate. So I think just do the brute force scaling, you can imagine a path forward. Right.
John Martinis:Obviously, if we can combine things, and we have some ideas on that, where we can combine things at the right place, I think we can do that too. But that's wrong time. But I would say the first thing we're doing is figuring out how to build a 20,000 qubit module with really good qubits. Very cool.
Sebastian Hassinger:So that brings us to the qubits. You've obviously got a track record in working in superconducting qubits, transmons. What can you tell me about the way you're designing the qubits for CoLab?
John Martinis:Okay, so this is a very complicated system engineering problem, and people have a lot of different qubits to do that. But getting the right control and the right crosstalk and whatever is difficult. So we think adjustable qubits, adjustable couplers is the way to go. Just this morning, I was listening to a very nice talk from a Chinese university who's been able to get this to work too. And their numbers are good, and Google's done that, other people have done that.
John Martinis:There's really some good reasons to think that that's the way to go. So it's that, and we think we have a design, we've done the theory, where we can make kind of the intrinsic errors, let's say the two qubit errors, that's the hardest thing, down in the 10 to the minus four range. If we get, if we can just get the coherence of like 200 microseconds, we think it could be in the three times 10 to minus four range. And that's including all the strays and everything. So Chris, that's on paper.
John Martinis:Yes. Paper qubits are the best.
Sebastian Hassinger:They are the best.
John Martinis:So we have to make physical qubits. We have a design in the refrigerator right now that we're testing. And, you know, various things look good. So, you know, but we're starting the whole process just saying, okay, we have to make really good qubits, and what is that design? What do we have to do?
John Martinis:We understand that, and we're going to focus on that. And then once we can get that to work, or a few qubits to work, then at the same time we're working on all the scaling so that hopefully we can do that relatively But there's a lot of control and software you have to build to do But that's the basic plan. We're not trying to build lots of qubits in the beginning. We're trying to build a small number but do it really well and build it with the future in mind. So, okay, so tunable coupling, tunable coupler, tunable
Sebastian Hassinger:transmon, tunable transmon laid out in a lattice.
John Martinis:Yeah, we think that surface code architecture is good. If someone comes up with some better ideas, we'll think about it. But coupling to a lot of qubits is hard. And you take, for example, this is what I've been told by some people at Google, you know, I don't, haven't done the analysis myself, but they do an architecture where sometimes you connect to two qubits and sometimes you connect to three. Right.
John Martinis:And, you know, that's really much easier to make something.
Sebastian Hassinger:Yeah.
John Martinis:But what I've been told is that if you have qubit dropouts, because we're not going to make a perfect chip, Right. Then that architecture is pretty intolerant to errors. Whereas surface code, can have, I think, one or 2% errors dropouts. And then you can work around it because the connectivity is So this is an example of a system engineering thinking that you can do things that is kind of simpler right now, but you really have to think long term, what's going to be more reliable. So reliability is a big Of course.
Sebastian Hassinger:When you look at over the last year, we've obviously had some really big stories around error correction. Yeah. And also, sort of new iterations of superconducting qubit design. So not just cat qubits, but dual rail qubits and, you know, the the, Atlantic qubit quantum circuits, etcetera. Do you see sort of that low level qubit design as being something that's somewhat flexible over time like in other words your your grand plan is is the the whole system architecture but you could potentially swap
John Martinis:out a different So our business plan is to get really good at fabrication. Okay. Okay and you'll do that well and we're gonna work it out for our own Cubic design. However, we're gonna generate the processes and PDKs and whatever so that if someone else wants to build their thing then they can build it in ours. In fact, we're meeting someone today or tomorrow to discuss, you know, make sure that we're going to come back.
Sebastian Hassinger:And that collaborative set of partners. It makes sense to have multiple potential qubit designs in that sort of large ecosystem.
John Martinis:That's straightforward to do. And the way that we designed the business model and our ecosystem, then we can change that. So someone has a better qubit, then that's fine. Now, what I would say is, although there's all these nice papers out there, and this is great, I really like it, we need to explore, it's not clear to me that they're going to have the right system design to get everything to work right. You know, for example, with our design, we think we can get a good, high fidelity qubit gate with the intrinsic fidelity in the 10 to the minus six or so.
John Martinis:So, you know, it's very, very good. With a thirty five nanosecond gate, a really fast gate, not quite as fast as the one qubit, but really fast. And given we have a limited coherence time, and you always want to make a fast gate, then that's a very good design. Good design, four qubits, you can lay it out. So we're willing to talk to people, but we think we have pretty good design.
John Martinis:But that's good. What you want to do is have a good baseline design, and if something better comes along, we'd be happy to pivot. And we'd be happy once we develop this, be happy to fabricate a chip for someone else so we can do that. We have no problem with that. Out of curiosity,
Sebastian Hassinger:so you're saying 200,000 potentially in one fridge, and your your your goal is sort of a million cubit system. Are you thinking about transduction at this stage?
John Martinis:So so there's there's three ways to scale to, you know, millions. One is to have a big fridge. A really big fridge. A really big fridge. Okay.
John Martinis:It's expensive, but yeah, it's a little bit ugly. It's not modular enough. Yeah. But, you know, okay. And then the couple of things has to do with transduction.
John Martinis:Everyone's talking about optical. Right. And the problem is it's not quite there yet. Right. But people are working on it.
John Martinis:And then we'd be happy if someone could develop Right. And the nice thing is building modules at 20,000 and then at, let's say, 200,000, it's very natural to think of those modules as the central CPU and the other modules as distillation for the T state, the A state generators, and then to network it in. So the surface code architecture allows you to build that modularity. But again, we have to make the optical communication. So people have gone a long way, but it's still, you know, It's one of the
Sebastian Hassinger:areas I watch with fascination Yes,
John Martinis:I know. I've learned a little bit about them, and I think people can do it, but the communication speeds are
Sebastian Hassinger:really I know, in hurts. Yeah
John Martinis:and then it's a lot of overhead and a lot of distillation you have to do so you know that that's a big research area so it we kind of have two different plans and then we'll see now also in the paper, our theory people have said you might be able to do kind of error mitigation and break up the system classically in a clever way so that you might be able to tolerate a lower, smaller system. So, you know, we'd be happy to consider that too. Obviously we can build systems available to us. But you know, right now we're at 100 qubits or so. Yeah.
John Martinis:And, it's hard to know what it's going to be like, you know, in the future.
Sebastian Hassinger:Yeah. I always think that about, you know, the, the, the speculation about algorithms or applications. It's sort of like, well, you have to sort of build it to see what you can build
John Martinis:it to the application, but we have
Sebastian Hassinger:to build. It's a chicken and egg problem. Yeah, that's right.
John Martinis:Standard problem we have to do that. So just to tell you a little bit about my talk. Yes, please. If you look at the rate of progress, let's say the Google Group the number of queues, it's a good proxy because they're making the queues better. If you extrapolate that out as to when we'll get to a million cubits, okay, I will be dead.
Sebastian Hassinger:I was guessing it was going be bad news.
John Martinis:That's bad news, okay. And what I also say is if you look at all the young people in the audience, they will be retired. And so we're in this situation now where we really want to make it go faster. Right. And that's the nice thing about a startup company is you're able to think more creatively, out of the box.
John Martinis:What do we need to do and try to assemble the team? You don't have that inertia. So yeah, we're basically for my personal goals is I want to
Sebastian Hassinger:Self motivation is good job.
John Martinis:Before I die. And I think we have a plan where we can rapidly accelerate it because first, you know, it's nice that this is funded well, both from private government, private industry and the like, but this long horizon is not good economically. Right. And we're trying to figure out how to speed it up.
Sebastian Hassinger:Capital has a limited attention.
John Martinis:That's right. That's right. And originally, when we did the quantum supremacy five years ago, it was ten years. Well, we're halfway through ten years, and we got a factor of two. So it's just a little slow.
John Martinis:And I'm going say that it's generically hard. So our position paper was, you know, what's an approach to solve that problem? And it's also approach, like I said before, is how to make it cost effective.
Sebastian Hassinger:Right.
John Martinis:And how to do it in a way that makes sense.
Sebastian Hassinger:I was thinking as well, know, I mean, again, from the sort of systems architecture kind of point of view, I mean, it makes sense that what we're chasing is that moment when we can leverage everything we've learned about CMOS. Right? I mean, that's the the most successful human scale story that's ever existed. We we fabricate, like, trillions of transistors a day at this point. So, I mean, in in some sense, does it feel like superconducting qubits are are, you know, one entering in a race, they sort of emerged around sort of February, but there's all of the qubits are kind of on that same race to try to get to the point where there's an inflection point where all of a sudden you can have that monolithic solution moment when suddenly you unlock the secret.
John Martinis:So so let me get you're you're right in what you're saying, but it's a little bit more nuanced, but it's an actually interesting thing. You want to take advantage of CMOS, but if you go to a CMOS foundry, and we actually tried that in the last few years, and we got a piece of paper out. Right. But the problem is they have CMOS tools and they want to shoehorn your process into a CMOS process. Yes.
John Martinis:Now for spin qubits, know, okay, you can do that, fine. But for superdang qubits, it's a little bit different of a process. So it's fine, but you still have to buy the tools and bring them up and all that. The way we figured this out, took us a while to figure this out profoundly, okay? We knew it intuitively from the beginning, is not to go to a foundry.
John Martinis:It's not a 300 millimeter foundry is the answer. Right, got it. You go to three hundred millimeter because they have the latest, most advanced tools. The latest processes are only in 300 millimeter and not in 200 millimeter. Right.
John Martinis:And if you want to back port them, I see. It's too expensive. Right. Because, you know, they don't want to redesign. There's no market.
John Martinis:Yeah. Okay. Yeah. And the other thing is we have to make two new tools, new process. We've got, okay, the process exists, but you have to combine it in a tool.
John Martinis:I see. So the secret is, is to go to a tool maker. I see. And in our case, that's Applied Materials. Got it.
John Martinis:Got it. That collaboration has been fantastic. Excellent. Okay. And it's basically because we can tell them what we understand about the physics.
John Martinis:And they go away and say, yeah, we have these Versa tools. And then we work together. And then we finally say, okay, these are the tool sets.
Sebastian Hassinger:So that's upstream from the fab, the eventual fab of
John Martinis:this. Yes. Because, and the thing is, is they know how to do the various processes and they have experience. And they all the time are selling to people who want to do something new and they have developed in labs, so they're used to this. And what we're doing is within what they're normally doing.
John Martinis:And the nice thing is the tools that we want to make this in are, let's say, 50 to $100,000,000,000 I don't know what it And I'm going to say that's even too expensive for Google or whatever until you're selling something. Right. It's way too expensive. But for them, they have these systems. They can retrofit it relatively cheaply and then try it out.
Sebastian Hassinger:I see.
John Martinis:And then they have a, you know, several billion dollar research clean room. Right. So for a modest amount of funding, working with the right people, and of course we have to convince them we knew
Sebastian Hassinger:what we were doing,
John Martinis:okay, fair enough, okay, Then you could take advantage of this.
Sebastian Hassinger:So as So it's tool, our tooling R and D process is what you're plugging into essentially. Yes,
John Martinis:that's right. And then they've been able, you know, they have to bring it up. It's taken a while to bring it up, you know, six months. Right. But they can bring it up in a way that just blows your mind in terms of like the university fab you ever because they have real experts there.
John Martinis:Yeah. And then also we're thinking about how to do certain things in a couple years, and they have experts on that too. Right. So you can really put together anything. But, you know, basically every process step is way better and way more reliable.
John Martinis:And the really interesting thing, and I'll be giving a talk on this later today, is we just make simple resonators, you know, okay, just to check out the materials. And that actually took a while to get that to work right, okay? But the reproducibility of those resonators, like the resonant frequency, is way, way better than we ever did. And that's over a huge 300 millimeter wafer. And you know, if you go look at the specs of what they're doing, they have nanometer precision on various things and flat surfaces and big brains and all these crazy things that you can't even imagine doing.
John Martinis:And that just comes out of, you know, their knowledge base.
Sebastian Hassinger:It's the transits, the spectrum from science, science done by postdocs and PhDs to engineering.
John Martinis:They have decades and decades of experience and then we take advantage of it. So it takes a while to get there, but then in the end, you have something that is really kind of amazing. Now of course we have to show that it's going to translate to My guess is what's going to happen is we're going to translate to something that kind of people have already done on their, let's say their best qubits or best But we'll be able to make it reliably. And we'll be able to make it over a big 300 millimeter wafer and get it with the parameters set right and get everything right. But there's a chance that it'll be much, because it'll be more reliable, it'll also be much better.
John Martinis:So we'll to check that.
Sebastian Hassinger:But it looks really, really interesting. That's exciting. So can you tell me when you'll have a system that you can start publishing results or getting people to kick the tires on?
John Martinis:Well, okay. So first of all, from the working with in Taiwan, Academia Syndicate, paper was posted yesterday on a process that gets rid of these liftoff junctions. And liftoff, of course, no one in their right mind in the semiconductor industry would ever do liftoff. It's very, very dirty. Maybe at the back end or something.
John Martinis:But to depend on that's bad. So we publish that. We still have to make it better. So that's published. We have the position paper.
John Martinis:And we are yeah, we're working on getting everything figured out. Now, one of the things, it's okay, it's gonna be better than publishing. Okay, we'll publish. Good. Okay.
John Martinis:What's better than publishing is that our CoLab business model is to make available to us our good qubits and our good system. The big thing we do is what's called the CoLab Start System, where we deliver a system that's essentially equivalent to our best research grade systems. So we take all the knowledge of that and we reproduce it and we give it to you or sell it to you because it's expensive. We sell it, and then people can use good coupons. And it's part of our collaboration strategy because we think university groups, they can test things that maybe we don't have the time with, or they're going to test and maybe come up with new ideas.
John Martinis:That's great. And then besides the qubit systems, we are going to make available chip mounts and some filters and some electronic testing so that you know people can make sure that their systems are kind of up the grade. So there's just a lot of infrastructure to make good qubits and we'll try to make that available.
Sebastian Hassinger:And when does that start start?
John Martinis:We're hoping actually last night we talked to a group and they said they wanted to buy a chip mount from us, hopefully in the next few weeks, And the co lab said there's another group that's talking about it, and we hope to get them out a quote and get a in the next month or so.
Sebastian Hassinger:Great, so this is going to be a big year for you then?
John Martinis:Well, if we can get all this to work, so that's good. And then, you know, as we make the chips and we've tested it enough for ourselves and maybe, you know, publish some papers, but it's going to be better because it's like the super appendix where you actually get a chip. Right. Okay. You know, that you can play with yourself.
Sebastian Hassinger:Right.
John Martinis:Right. And then I would say one of the things we're trying to do is to make these good qubit systems requires a lot of patience and calibration and the like. And we're working closely with quantum machines to do that right, make sure the control systems are good and doing that. But we're trying to fix various things in the CUBA chips at the low level so that when you go to calibrate it, it's a lot going to be a lot easier. And, you know, because what we did at UCSB and then Google really required, you know, a race car driver to drive the chip.
John Martinis:We wanted to be, you know, not quite a Tesla self driving, full self driving, but you know, towards that, we want
Sebastian Hassinger:to move in that direction. I mean, Tesla's just moving towards that as
John Martinis:well. There's a
Sebastian Hassinger:lot of But you
John Martinis:understand, we want to move towards that. And it's not just making better software, you want to make better hardware. As an example, we're collaborating with Synopsys, who makes EM simulation design And we're working with them to figure out how to do an EM simulation on, you know, a thousand qubit device or something. It's actually a hard problem because of the different length scales involved. Right.
John Martinis:And, but they have, some knowledge base and they've done it on other kind of problems and they just have to apply it
Sebastian Hassinger:to That's fantastic John, I think it sounds very much like you found a model that is going to have sort of multiplying positive effects on the whole ecosystem because everybody who's involved in that partnership.
John Martinis:Yeah, and okay, we're gonna help other people make money and that's fine, okay. I'm just excited. We wanna have a valuable company, we think we'll do that. We think that's the way to it. And I think we have to do this because I think the physicists are being a little bit naive what it takes.
John Martinis:I don't know exactly what everyone's doing. But I think a horizontally integrated, a lot of collaboration is gonna help
Sebastian Hassinger:the field a That's fantastic. Well, we'll be watching
John Martinis:Oh, and by the way, it's really fun. Of course. And because I get to talk people who are experts in their domain, And then we get to learn about all these things in great detail. Right. You know, in a way that you could never hire someone in your own company.
John Martinis:Of course. With that kind of expertise. Yeah. And then also I find is talking to the managers of the other companies, they're really good and they're used to managing a hardware project, which is different than managing a software project. Right.
John Martinis:And that's, you know, at least at our level, that's fine. And it's really interesting to learn from them. So, yeah, I get to get to experience things, which I find really, really fun.
Sebastian Hassinger:It matches with, I mean, tend to have a very holistic perspective and that's the approach that you found with Colab. So it sounds Yeah. That's right. Terrific. Thank you so much for joining us.
Sebastian Hassinger:This has been great. Excellent. Great. That was fun. Thank you for listening to another episode of the podcast, which is a production of the New Quantum Era hosted by me, Sebastian Hassinger, with theme music by Omar Costa Hamidou.
Sebastian Hassinger:You can find past episodes on www.newquantumera.com or on blue sky at new quantum era dot com, where if you comment on or reply to a post about an episode, your comment will go straight to the website, so we'll be sure to see it and respond. Thanks again to the APS and Quantum Machines for their support of this episode, and thanks to John Martinez for a really enjoyable conversation. If you enjoy the podcast, please subscribe and tell your Quantum curious friends to give it a listen.
Creators and Guests
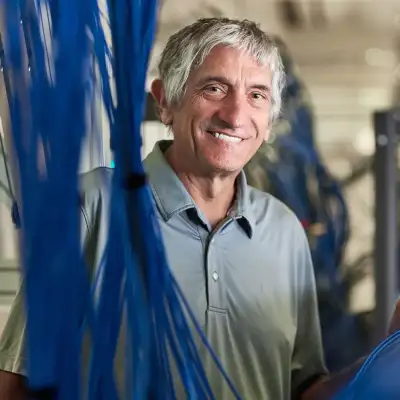
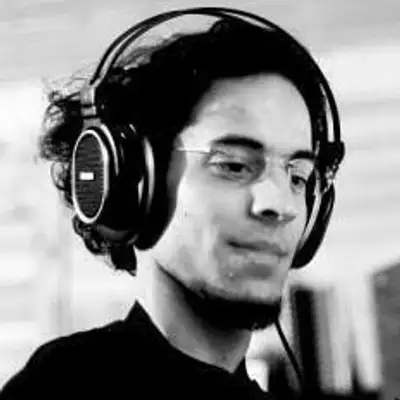