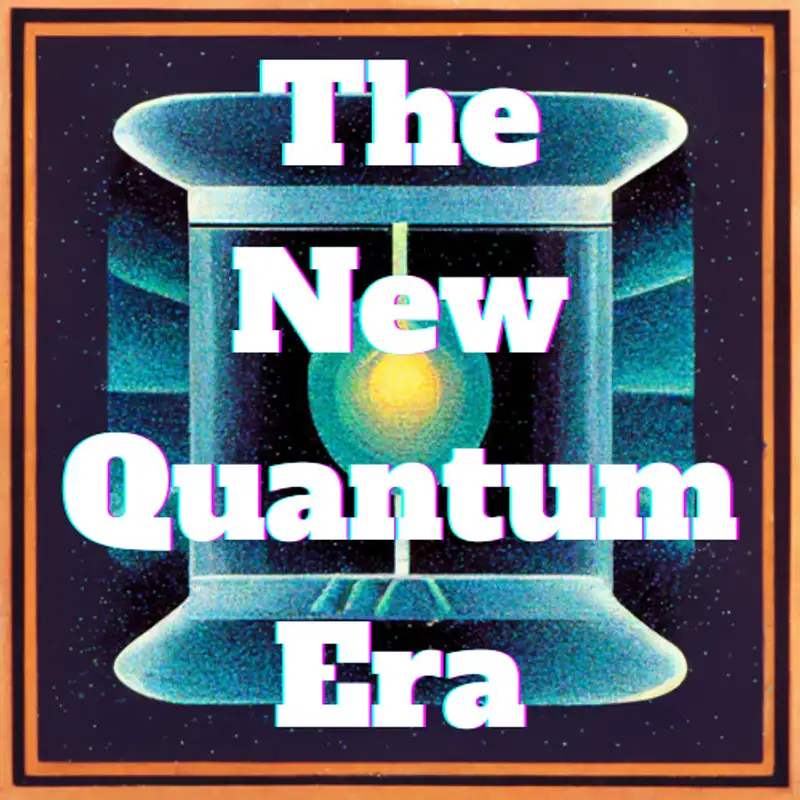
Informationally complete measurement and dual-rail qubits with Guillermo García-Pérez and Sean Weinberg
This is the new quantum era, and I'm your host, Sebastian Hassinger. Before we get into the episode, a few administrative notes. Repeat listeners will have noticed a different opening. I've done away with the prerecorded opening that Kevin and I have used for the last few years. This does not mean that Kevin is not part of the podcast.
Sebastian Hassinger:He will be joining me as a cohost when his schedule allows. But I'm planning a lot of in person episodes, and those are particularly difficult to, coordinate with our respective schedules. And so we decided it was time to make some changes to the way the podcast sounds when you listen to it on your podcast app. Other than that, though, the format of the show remains the same. We'll be interviewing researchers in the field of quantum computing about their work, and today's episode is no exception.
Sebastian Hassinger:It's a a little bit of a departure for us because we have representatives from two different companies that are collaborating. They're both from an academic background. Sean Weinberg is the director of quantum applications for Quantum Circuits Incorporated, recently spun out from Yale University. And Guillermo Garcia Perez is the chief science officer for Algorithmiq, a European software company that is focusing on quantum algorithms, and they were founded by Sabrina Moniscalco and her postdocs and PhD students, from the University of Helsinki. So we'll be talking about their collaboration, which is looking at a new technique, a new algorithm for ground state simulations using the forthcoming Aqumen Seeker device that Quantum Circuits Incorporated is launching this year.
Sebastian Hassinger:So have a listen. Welcome back to the podcast. I'm joined today by two special guests from two different companies. It's kind of a new thing for the podcast. We're gonna have an interview with, new partners, which is quite exciting.
Sebastian Hassinger:So with us today, we have Sean Weinberg, director of quantum applications from Quantum Circuits Incorporated, and Guillermo Garcia Perez from Algorithmiq, the chief chief science officer. Algorithmiq is a a a quantum software company focused on, pharma and chemistry in particular. They've focused on on algorithm design. They've done a lot of really innovative work. And, Quantum Circuits, of course, is a hardware company spun out of Yale.
Sebastian Hassinger:We talked to Rob Schoelkopf, who's one of the founders, just a few weeks ago, and we're we're really excited to be joined by Sean and by Guillermo. Welcome, guys. Thank you for joining.
Sean Weinberg:Thanks for having us.
Guillermo Garcia-Perez:Thank you.
Sebastian Hassinger:Absolutely. So so your your respective companies put out a joint press release just a couple days ago, that was quite exciting. Of course, Quantum Circuits is planning to make a device available, in the near future. That's called the Aqumen Seeker system. Right, Sean?
Sean Weinberg:Yeah. That's that's correct. The Seeker.
Sebastian Hassinger:Excellent. And so the press release was about essentially a new partnership between the two companies. Guillermo, can you tell me a little bit about what that partnership's about?
Guillermo Garcia-Perez:Yes. Yes. Of course. So, as you probably know, so, QCI has, dual rail qubits, which is, a very interesting technology because it has built in error detection. And, our algorithms and I'm I'm very happy to give you more detail on that.
Guillermo Garcia-Perez:Our algorithms are very comfortable, let's say, with, dealing with noisy computers. But if we can kind of filter out, what we know, like, the runs that we know that are affected by errors, then it makes our life much easier. So the key point of our collaboration of our partnership is, at the moment, is exploring how error detection can, essentially make things much better for our for our algorithms and how we can extract a quantum value, let's say, from from these devices and our
Sebastian Hassinger:Very cool. Yeah. I know. I mean, prior work by Algorithmiq with IBM, I remember, was a a variational technique. It was an approach where the more iterations you did, the the lower the error rate.
Sebastian Hassinger:You're actually getting more and more accurate results. This is a couple years back. And then more recently, you guys launched a a a tensor, error mitigation, approach. Right? Is is this, work that you're planning on the seeker devices that sort of extrapolating from that prior work, or is this novel?
Guillermo Garcia-Perez:That that's a very good question. Is it is partly related in that the, at the very core of, the algorithms we use, there is something we call informationally complete measurements. So, basically, that's we use a very specific way of measuring that allow us to do something that's very useful, which is we can just measure on the device, and then the data we we obtain can be basically fed to a a classical method that can post process it. And the that work you're referring to, the this very written one, which we used, tension network error mitigation, The idea is that then you have a 10 to network method that kind of cancels the noise that, affected the circuit execution. What we're doing now is related to in related to that in the in that we also measure with information complete measurements, and we feed it to a tensor network method.
Guillermo Garcia-Perez:But the tensor network method we use now is different. It's variational, which means that, basically, we optimize in post processing, in order to reduce the error sorry, the, the the energy the energy of the system was simulating. And this is a very novel approach that we've, we've developed, in the last several months, let's say, we're very excited about because one of the key elements of this of this algorithm is that if the data we get from the quantum computer imagine something goes wrong and you get just basically random numbers, which is there's no advantage there, then the algorithm is going to be at least as good as one of the state of the art, methods in computational chemistry. So there's no disadvantage in that sense. It's going to be at least as good as that.
Guillermo Garcia-Perez:But if you get a good quality state from the quantum computer and therefore the data you use is high quality, then you can do much, much better than you could just classically. And that's precisely one of the key points that we want to prove, in our collaboration as well. And we've we've seen traits of that in in our current you know, in our experiments together so far. We've seen that, there is an advantage in, taking the data that has has been, error dissected. So the one that we've postselected, the the the data we know that has didn't have the most significant errors, and then we fed that to the algorithm, we could see an advantage, with respect to the non error detected data or the complete complete random random output.
Sebastian Hassinger:That's very cool. And and, Sean, as a as a somebody looking at quantum applications in a hardware company, what what do you see as the the main sort of purposes of this type of early collaboration with with, a company like Algorithmiq?
Sean Weinberg:Yeah. That's a great question. So, there's there's many aspects to it, but what I would highlight is, first of all, I think it's fairly well, understood, in in, you know, among the people that think about applications of of quantum computing that applications in in, chemistry, which includes pharmaceuticals and also material science, anything that where where you're basically trying to use a quantum computer to get a better understanding of a physical quantum mechanical process. These are the applications that may be the most near term, and there's good reason for that. So, we're starting with an area that we already have higher confidence in when it comes to can we get something useful without waiting ten years.
Sean Weinberg:But as we've seen, in the past five or so years in the industry, that doesn't really solve your problem, okay, because we don't have fault tolerant quantum computers.
Sebastian Hassinger:Right.
Sean Weinberg:So, you know, it's been it's been, it's been kind of the key challenge, in the quantum computing industry to figure out, you know, how can we make some value with these devices, without waiting so long. Now we are trying as hard as we possibly can to accelerate that process. And this this collaboration with Algorithmiq, is is really an example of that. And let me let me kind of explain why. So first of all, the application area is one of the ones that's, you know, relatively near term.
Sean Weinberg:Second of all, Algorithmiq is using a hybrid algorithm, which as as, as Guillermo mentioned, by default, is is going to perform at least as well as a state of the art classical method. And then data from the quantum computer is basically used as additional input to accelerate the algorithm.
Sebastian Hassinger:Right.
Sean Weinberg:So we've got we've got that, which sounds which sounds very nice. But now comes kind of the missing ingredient, which is, okay, where is that data actually coming from in the first place? And the answer is, in our case, it's coming from our dual rail quantum computer, which is which is Seeker currently. But but, you know, more generally, we're talking about using a dual rail system. Now our dual rail cavity qubit, you know, as as was discussed when you when you talked with Rob in the earlier episode, it's basically, it's basically a quantum computer where individual qubits have the ability to flag their own errors.
Sean Weinberg:They don't flag all of their errors, but they flag 90% of them, which is fantastic. Yeah. And, you know, basically, what you're getting from this is you are, sort of taking a step toward fault tolerance without quite having fault tolerance. Right? It's it's it gets you kind of one step closer to it, which which has multiple benefits.
Sean Weinberg:In the near term, it gives you basically a huge fidelity boost. Right. And in the long term, it makes it easier to follow that row there correction. Right. So okay.
Sean Weinberg:So so if you put all these pieces together, what we're really doing is we're just trying to, like, align as many stars as possible to do something useful with a quantum computer in less time. You know, we've got a good application. We've got a hybrid solver, and we've got error detection built into the hardware, and we're gonna see if we can get a more useful result with that.
Sebastian Hassinger:Right. Right. I suppose also in in very simplistic terms, as a hardware company with a brand new device sort of out of a university lab and into a beginnings of a commercial product, you want sort of the bet most qualified users to kinda stress test your your technology, right, and find the Yeah. The ways in which you can get the best performance out of it and and improve the design iteratively as you move forward.
Sean Weinberg:That that's right. That's right. I mean I mean, look. Like, Quantum Circuits is, a company which is absolutely devoted to designing the best possible quantum computer for the goal of getting the fault tolerance. We need to work with unbelievably good partners that are going to design algorithms, that that we can then run on the system.
Sean Weinberg:We have this alpha user program, which which is basically, you know, we are going to early users, alpha users, giving them access to our system. And, you know, these alpha users are, teams that, you know, have a immense amount of expertise in their area, and find ways to make the most of our system. And that's what Algorithmiq is doing. Algorithmiq has has, you know, unbelievable, technology with their, with their, solvers sorry, with their, you know well, Guillermo can explain it much better than I can, so I'm I'm not gonna try to, follow my words here. But, you know, that that's that's kind of the point.
Sean Weinberg:Right? We we we work with partners that are going to find better ways to use our system. And, you know, for example, Dheermo, maybe you maybe you can comment on this, but we might have the ability, for example, you know, quantum circuits has the hardware, Algorithmiq has the algorithms, and then we go to an end user together
Sebastian Hassinger:Right.
Sean Weinberg:That will, you know, actually use the kind of full system, the full workflow that we together are building here.
Guillermo Garcia-Perez:Yes. Absolutely. This is this is something we're very interested in because, at Algorithmiq, we've always thought about, delivering value for real use cases, not just simulating chemistry for the sake of it. It has to be real use cases. And in fact, everything we've been, testing so far has always been on on things that are biologically relevant, including, for for instance, the our collaboration so far.
Guillermo Garcia-Perez:What we've been working on is related to drug metabolism and things that matter for the industry as well. So Right. But having an end user, that tells that comes to us with a specific problem that they find relevant, that would be very, very interesting. That's great.
Sebastian Hassinger:And and and so just sorry to interrupt, Guillermo, but I just wanted to make sure I'm clear on one point from your introduction, and then what what Sean was saying. So Mhmm. What you're saying is that, I mean, the sort of, the the known variational quantum eigensolver pattern for a hybrid, algorithm takes a classical code to create an ansatz, which is sort of your first approximate guess, throws it at the quantum device, and, takes the result to refine the, the the the the approximation, then iterates back and forth. But are you saying that you're starting with a quantum circuit that you're running on Seeker and then feeding that result into a classical variational solver? Okay.
Sebastian Hassinger:So so the classical variational solver is, you know, bread and butter in in chemistry simulations to a certain degree. I'm sure you've got a special version of it, but it's a it's a known approach. And as you were saying, so the the classical solver has sort of a a baseline, which is the performance you expect from from that solution today. And what you're doing is injecting a quantum result, a quantum computing result into the beginning of that variational process that would, does it increase the accuracy, or is it reduce the time to get to the approximate the final approximation? Or both?
Guillermo Garcia-Perez:So, these are very, very deep questions, and I you'll probably have to stop me at some point because I can just go
Sean Weinberg:for a while now.
Sebastian Hassinger:Well, we'll run out of time at some point, Kierma, but Yeah. Absolutely.
Guillermo Garcia-Perez:I'm trying to be very so there are two main so you've touched on two things that, I'd like to clarify a bit. I'd like to say something about both of them. So I'll start with the the post processing part, because it's the the thing we were discussing earlier. Yeah. So the algorithm is precisely as you said, it's an algorithm that runs variationally.
Guillermo Garcia-Perez:So what's variationally, in this case, what I'm referring to I was referring to, is the post processing part. So we take the state, as you said, like, let's call it a reference state. So you have a circuit that you've obtained somehow. You're running it in Seeker. You measure with information and complete measurements, and that gives you, classical data that then you can work with in post processing.
Guillermo Garcia-Perez:And we feed that data to, this, tensor network method that basically tries to minimize the energy of that outcome.
Sebastian Hassinger:Okay.
Guillermo Garcia-Perez:So with information and complete measurements, what you have is that, basically, after you've measured, you can decide what quantity you want to evaluate, which is very different from how things are normally done because here you after you've measured, you decide, I want to focus on the energy. And then you can then, use a tensor network to transform that kind of state you have or representation of the state you have. And that tensor network can be optimized after you've used the quantum computer. So you don't have to touch the quantum computer again at at that point. Right?
Guillermo Garcia-Perez:But, of course, what you want is for that data you're feeding to that algorithm to be as good as possible. And that's where, for instance, error detection plays a crucial role. Because if you don't have error detection, you can still use all the data. So Yeah. Not having error detection means you know that in your data, you have outcomes that come from runs where there's been a significant error, but you just don't know which of those Right.
Guillermo Garcia-Perez:Are are basically read a little bit error. So you have to deal with all of that. And that means classically, your classical computer has to handle huge amounts of data. Right. And the quality of that data, because it's the average of data with without significant errors and data with significant errors, it's not as good.
Guillermo Garcia-Perez:But you can still do it. It's just less likely to be, advantageous, let's say. Right. If you have error detection, you can just basically look at those flags and then throw out the data that you know it's it's wrong. And then you just use the rest of it, which still has some errors, but as as Sean said, but they're not that significant.
Sebastian Hassinger:Right.
Guillermo Garcia-Perez:And then that classical algorithm basically uses that data as an input, and it's not so much about the time. It's I mean, it's always about the time in a way because you can always rewrite algorithms in a way that it's just getting to that is just takes longer. But let's say the thing is that the you can think of it in terms of solutions you can access. Mhmm. Mhmm.
Guillermo Garcia-Perez:With a quantum compute like a perfect quantum computer, you can, basically, entangle the qubits to a degree that you can't do you can't simulate classically. So that's a that's a resource. Right? If you have some noise, you can still maybe entangle, correlate the qubits a lot, but it's it doesn't mean that you can perfectly prepare, say, the ground state of a molecule because of is right. But the the work it takes to, say, correct for that, for the effect of noise, classically, might be much, much smaller than the the time it takes to actually simulate the state from from the get go, from from scratch.
Guillermo Garcia-Perez:And that's that's the the the philosophy here. And that's that's for that part of the of the algorithm, just the second part, just given the reference date of what we do, afterwards. And if if I may now, I would like to jump
Sebastian Hassinger:Yes.
Guillermo Garcia-Perez:Onto the other part, which we mentioned, is like the bread and butter of computational chemistry on on computers, which is variational, methods, like variation of quantum mechanics. That's indeed, a very good approach in that you don't so you have to know given the problem once you've transformed the problem into something that makes sense. So you know, exactly what you you you you're after. You have your your system set up. You still have to find, the circuit or the sequence of operations that you have to execute physically on the device in order to simulate the the state that you care about.
Guillermo Garcia-Perez:And what the variational quantum eigensolver does is it seeks that state, in a kind of feedback routine where there's a bit of back and forth. The problem with that is that there's many challenges with with that specific, approach because you have to optimize a circuit whilst using the hardware, and that's very costly because hardware is it it takes a while to run. You know, to you you can't just extract information exactly. You can you have to measure, and that means you have statistical errors. Right.
Guillermo Garcia-Perez:It makes the whole thing not very practical in practice. And, also, there's noise. There's always Yeah. For now, for the for the time being and for for the next few years, there's going to be at least some levels of noise. So that makes the optimization of the circuit very, very so obtaining just a circuit that approximates the ground state or the excited state, the state you care about, it it makes it very challenging.
Guillermo Garcia-Perez:Mhmm. And our take in, at Algorithmiq is actually quite different from that. So we still go for a, say, a a variational method that optimizes a circuit, in order to get a good reference state, but we don't touch a quantum computer for that. So we've developed a set of algorithms that when combined together, allows us to get a very good reference state even at very large scales before we even touch a quantum computer. And that's a very key part of, very key innovation Right.
Guillermo Garcia-Perez:For the fields because that's a problem even in the fault tolerant era. In the fault tolerant era, there's things like
Sebastian Hassinger:I was gonna say people often sort of skim over the, you know, imagine a a large fault tolerant quantum quantum computer, you still have the problem of classical overheads in state preparation and post processing. So, like, all three of those stages, so to speak, are going to need the kind of innovative work that you're doing as a as a as partners here. Sean, this the the the seeker device is a a small number of qubits. I I think it is it is it I can't remember the number now. I've forgotten.
Sean Weinberg:It's eight on the seeker.
Sebastian Hassinger:Eight. That's what I thought. That's right. So so eight, dual rail qubits with, 90% of the errors are flagged. So you've got quite a bit higher quality output, than let's call it a, you know, a, an a simple NISQ device.
Sebastian Hassinger:It's sort of an enhanced NISQ device in a sense. Do you foresee you know, the the scale of the device means I assume that they'll be, you know, relatively, there'll be limits to what the the results can be from from this use by Algorithmiq, just because of the scale of the machine, do you think that over time, you'll evolve the devices to be larger numbers of qubits that still with sort of the error mitigation that the the, the the noise bias, the 90% flagging of errors, or, is is it sort of the next stage just to implement error correction and get to fault tolerance at a small number of qubits and then scale up beyond that?
Sean Weinberg:Yeah. Okay. Good questions. Okay. So first of all, the seeker proves that the dual rail concept works perfectly.
Sean Weinberg:It works as a system. We can deal with all kinds of, you know, issues that often arise in quantum computing systems. We've been able to successfully deal with them. So we know now that the architecture works. Now it's just a matter of scaling it up.
Sean Weinberg:That's our point of view. Now you asked a few questions about scale up both from the point of view of, are we gonna continue to flag errors with the same kind of efficiency and use it in algorithms like what Algorithmiq is doing? The short answer is absolutely yes. And then, you also asked about are we gonna start having to really start correcting errors, correcting including the detected errors and any other types of errors? And the short answer to that is, of course, yes.
Sean Weinberg:But let me let me try to give, slightly better answers here. So, first of all, the whole point of the dual rail architecture or the kind of initial point, what does a what does a dual rail qubit do that's special? Maybe this is a little bit redundant with your discussion with with Rob, but I'll be I'll be quick.
Sebastian Hassinger:That's fine.
Sean Weinberg:I'll try to be quick. It's actually pretty easy to understand the dual rail qubit. If if you're someone who's a little bit new to quantum computing, and you're listening to this, a dual real qubit you can use just like a conventional qubit. It has a zero state, it has a one state. Now the trick is that the way that we encode the zero state and the one state, we put the the zero state is basically we put an excitation on one side of the dual rail, and a one state we put an excitation on the other side.
Sean Weinberg:So it's actually completely symmetrical between the zero and one state. Now this has a little trick, which is that if the excitation is not on the left or the right, then there must have been a problem. And we have the ability to detect when that happens. Right. And that detection says, hey.
Sean Weinberg:There was a problem on qubit number five. Turns out that gives you a huge, a huge number of advantages, that that are some of them are a little bit technical to discuss related to, what we call hierarchy of errors, which is basically the statement that the most common error is this detectable one. And then after that, there's dephasing, and then at a much, much lower rate, there's bit flips. Right.
Sebastian Hassinger:But This is erasure is what you're talking about. Right?
Sean Weinberg:That's right. Yes.
Sebastian Hassinger:Okay. Or just
Sean Weinberg:what you're saying. Precisely. So the term erasure is is, you know, a very interesting a very well chosen term, actually, but it may surprise people to hear it. Erasure means an error at a specific location when you know the location. And you might say, that doesn't sound like what an erasure is.
Sean Weinberg:But if you draw some zeros and ones on a sheet of paper and I take an eraser and erase one of them, you know which bit is missing because you can see the smudge. And that's kind of what we're talking about. Okay. So, as we scale this thing up, which we are absolutely doing, and, you know, we we intend to do this, you know, in the near term, with, with partners such as Algorithmiq, and we continue to intend to run algorithms where we're going to just, you know, flag erasures and plug things into a, you know, an algorithm workflow like what, Guillermo just discussed. Now you might ask questions like, okay, well, how is this gonna work as as it scales up, you know, with the ratios popping up kind of all over the place if you have, like, you know, 50, of these qubits.
Sean Weinberg:And and and it's a it's a fascinating question, but I I wanna kind of impart what I think is a very important insight, which is that, all qubits have errors. All these NIST devices have errors. The difference is we know where 90% of the errors are. That's the only difference. So it can only be a good thing.
Sean Weinberg:Now the only technicality on that is, like, what are the error rates? And of course, we are constantly making advancements in the lab on getting our error rates down.
Sebastian Hassinger:Right.
Sean Weinberg:So we we are really excited about this about this new approach. Okay. Now I I have to make just one comment about error correction, and I'll I'll I'll make it quick, which is that, if you know where errors are, it's a lot easier to correct them.
Sebastian Hassinger:Yes.
Sean Weinberg:And that is kind of the heart of why the erasure error is is really beautiful.
Sebastian Hassinger:Yeah. I mean, what excites me about your architecture is that it's you know, I see it as sort of a part of a new generation of hardware that is trying to, as you said, limit the scope of the problem of error correction, instead of, you know, having to create these very high ratio physical to logical qubit architectures because you can't assume anything about where and when the errors are gonna occur or how they're gonna occur. You're creating something that that, sort of almost forces errors into a narrower scope, which makes it, I I assume, a lower ratio of of, sort of overhead, to get to that corrected sort of logical Qbit outcome. Right?
Sean Weinberg:Yeah. Yeah. That that is definitely correct. What you just what you just said is is is a very nice way to put it on the error correction side. Now on the near term algorithm side
Sebastian Hassinger:Right.
Sean Weinberg:Like what we're exploring with with Algorithmiq, actually, the the same logic can apply. If you think about what what people have been trying to do with error mitigation for a while, you know, it all comes down to we've got this data coming from the quantum computer. We know there's problems, and we need to do something smart, you know, in lieu of error correction
Sebastian Hassinger:Right. To get
Sean Weinberg:a good result. Extraction. Yeah. Signal extraction. Exactly.
Sean Weinberg:So Yeah. So it it can't hurt if you know where the locations of the errors are. And I think there's a a very wide space of, you know, algorithm exploration. And I think we're starting that with Algorithmiq. But but, you know, there's still a pretty wide universe of algorithm exploration that can leverage this additional information.
Sean Weinberg:It's like, you know, information has to be useful.
Sebastian Hassinger:Yeah. Yeah. And and Guillermo, I mean, from your perspective, if you were if you had the reins on the, the direction that QuantumCircuits was going with their next device, given your approach, would you prefer a larger number of of, dual rail, you know, the, qubits that you could then air mitigate and extract signal from, or would you prefer that they nailed error correction and gave you logical qubits even if the the overall number was smaller?
Guillermo Garcia-Perez:That's a very good question. I think in the long run, I would be I'd listen to this to the experimentalists and whatever they think the best way
Sebastian Hassinger:of getting a better way to evaluate.
Guillermo Garcia-Perez:Yeah. I, I, it's, it's a difficult question to answer because I think both things are extremely exciting to explore. And, of course, yeah, the the idea of having an error corrected computer, even if it's smaller than a noisy, larger, larger scale computer, both things are very interesting because even in the second case, like the noisy computer would still have a red section. And as Sean said, like, the the way we're using right now, a red section is basically step zero, which is the the most naive thing you can do is Right. Know there's been an error here.
Guillermo Garcia-Perez:Just throw it out. But there's more we can do because with, dual rail qubits, you can also tell when the error happened. You can Right. Kind of monitor, when the error happened. So we could probably do something cleverer than that.
Guillermo Garcia-Perez:It's just, something that we haven't yet
Sebastian Hassinger:That's interesting. So So potentially using, like, partial results or or using, like, interim results before the error occurred as as part of your solution potentially?
Guillermo Garcia-Perez:Sorry. Could could you repeat that?
Sebastian Hassinger:Oh, I'm just curious. Like, if you're saying that, you know, when and where the error occurred, would you be able to potentially extract value from the the partial results before that error occurred?
Guillermo Garcia-Perez:Yeah. Definitely. Yeah. Interesting. I don't have a very concrete way to to to to answer that question, but we believe that's the case.
Guillermo Garcia-Perez:Yes. Still, as I said, it's still early stages of that sort of ideas, but we've been thinking about them. Yes.
Sebastian Hassinger:That's fascinating. Well Yeah. This has been really interesting. I I think what's, most sort of stimulating about this is that I haven't had a conversation, certainly on the podcast, where I've it's been so apparent that, you know, the the historical collaboration between theorist and experimentalist in physics itself is carrying over into the development of this, you know, new technology and new market around the technology. I think that's really, really fascinating.
Sebastian Hassinger:I think that, you know, that's one of the things I find the most exciting as I've, you know, sort of gotten to know the physics community that's working on the the the foundations of the beginnings of this technology. And it's really great to see it sort of carrying on into the, continued development of the technology. So, I thank you both very much for joining me, and I'm going to be watching very closely as you continue your collaboration, your experimental results. Thank you very much. The podcast is a production of the new quantum era.
Sebastian Hassinger:It is produced and edited by me, Sebastian Hassinger, with the music by Omar Costa Amido. If you enjoy the podcast, please subscribe and share with your friends. They can go to newquantumera.com to access the podcast, and also the book that I'm writing on O'Reilly's Riley's site is available, the first twelve chapters as a preview. Thank you, and we'll look forward to the next episode.
Creators and Guests
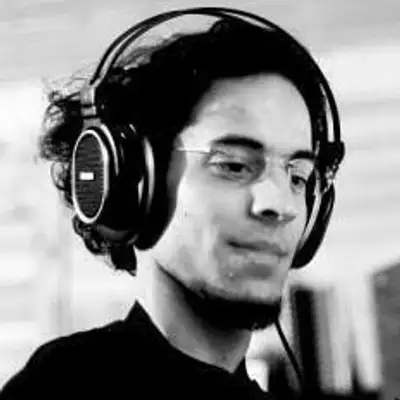